Penerapan Metode Naive Bayes Untuk Pengelolaan Status Retensi Arsip
DOI:
https://doi.org/10.36350/jbs.v15i1.279Keywords:
Accuracy, Archives Management, Classification, Naïve Bayes, PrototypeAbstract
Archival management is an important aspect of information management in various institutions, one of which is determining the retention status of archives. Determining the retention status of archives is still not accurate and effective, so there is a possibility of human error or inaccurate errors when determining the retention status of archives. One method that is appropriate for the problem is the classification method. The classification method used is Naive Bayes which will help archive operators. The purpose of this research is to classify the retention status of archives and create an application prototype. This approach allows archive managers to make more informed and efficient decisions about how to store or destroy documents, so they can reduce the risk of errors and optimize the use of storage space. The variables used are archive type, index type, active retention time, in-active retention time and class predictor, which is the archive retention status. Prototype application to determine archive retention status using the Naive Bayes Algorithm method. The test results using confusion matrix showed an accuracy of 96%, the accuracy of the user test questionnaire results was 100%, and the accuracy of the system expert test questionnaire results was 92.86%.
Downloads
References
[1] U. Gellysa, Penerapan Data Mining DI BERBAGAI BIDANG, 1st ed. Bali: SONPEDIA, 2023.
[2] Amna, Data mining, 1st ed., vol. 2, no. January 2013. Padang: PT GLOBAL EKSEKUTIF TEKNOLOGI, 2023.
[3] Y. S. Hidayat, A. G. Herdipriyansah, I. A. Agustin, J. Jaenudin, and D. Desrizal, “Pemodelan Data Mining Menggunakan Neural Network Untuk Seleksi Mahasiswa Penerima Beasiswa Bantuan,” TeknoIS: Jurnal Ilmiah Teknologi Informasi dan Sains, vol. 14, no. 1, pp. 9–20, Jan. 2024, doi: 10.36350/jbs.v14i1.222.
[4] J. W. Iskandar and Y. Nataliani, “Perbandingan Naïve Bayes, SVM, dan k-NN untuk Analisis Sentimen Gadget Berbasis Aspek,” Jurnal RESTI (Rekayasa Sistem dan Teknologi Informasi), vol. 5, no. 6, pp. 1120–1126, 2021, doi: 10.29207/resti.v5i6.3588.
[5] Alfandi Safira and F. N. Hasan, “Analisis Sentimen Masyarakat Terhadap Paylater Menggunakan Metode Naive Bayes Classifier,” ZONAsi: Jurnal Sistem Informasi, vol. 5, no. 1, pp. 59–70, 2023, doi: 10.31849/zn.v5i1.12856.
[6] F. Gorunescu, Data Mining, vol. 12. Berlin, Heidelberg: Springer Berlin Heidelberg, 2011. doi: 10.1007/978-3-642-19721-5.
[7] I. Kasim, Yuyun, and S. Sahibu, “KLASIFIKASI SURAT DIGITAL MENGGUNAKAN ALGORITMA NAÏVE BAYES,” JURNAL IT Media Informasi IT STMIK Handayani, vol. 13, no. 2, pp. 57–62, Aug. 2020.
[8] A. Triawan and D. Lintang Melinda, “Penerapan Metode Naïve Bayes Untuk Rekomendasi Topik Tugas Akhir Berdasarkan Daftar Hasil Studi Mahasiswa di Perguruan Tinggi,” Teknois: Jurnal Ilmiah Teknologi Informasi dan Sains, vol. 10, no. 2, pp. 58–70, Nov. 2020, doi: 10.36350/jbs.v10i2.91.
[9] F. Septianingrum and A. S. Y. Irawan, “Metode Seleksi Fitur Untuk Klasifikasi Sentimen Menggunakan Algoritma Naive Bayes: Sebuah Literature Review,” Jurnal Media Informatika Budidarma, vol. 5, no. 3, p. 799, 2021, doi: 10.30865/mib.v5i3.2983.
[10] S. Arikunto, Prosedur Penelitian Suatu Pendekatan Praktik. Jakarta: PT. Bina Aksara, 2010.
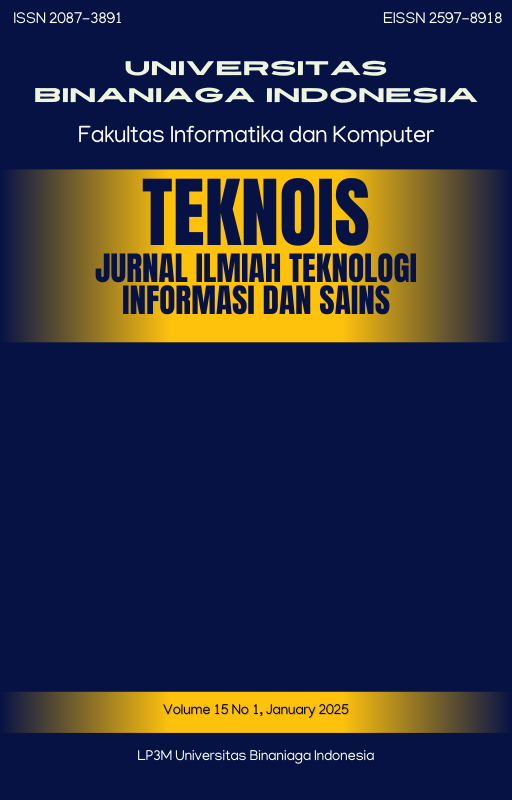
Downloads
Published
Issue
Section
License
Copyright (c) 2025 TeknoIS : Jurnal Ilmiah Teknologi Informasi dan Sains

This work is licensed under a Creative Commons Attribution-NonCommercial-ShareAlike 4.0 International License.